Learn how to maximize the performance of ChatGPT with the ultimate ChatGPT prompt engineering guide. Discover the importance of prompt engineering, tips for effective prompt crafting, and best practices for continuous improvement. Enhance your results with ChatGPT today.
Introduction
Importance of prompt engineering in maximizing ChatGPT
In order to get the best results from ChatGPT, it is essential to carefully craft the prompts that are fed into the model. The quality of the input prompts directly affects the quality of the outputs generated by ChatGPT. Prompt engineering involves considering various factors, such as context, input length, and user tone, to ensure that the model is receiving the most effective prompts possible.
Purpose of the article
This article aims to provide a comprehensive guide on prompt engineering for ChatGPT. It covers key concepts and factors that impact the performance of ChatGPT, as well as techniques and best practices for crafting effective prompts. By following the guidelines outlined in this article, you can maximize the performance of ChatGPT and get the most out of this powerful language model.
Understanding ChatGPT
Brief overview of its architecture and training data
ChatGPT uses a transformer-based architecture, which allows it to handle sequential data, such as text, with high accuracy. The model has been trained on a massive corpus of diverse text data from the internet, including books, articles, and conversation logs. This training process has allowed ChatGPT to learn patterns in language and generate highly coherent responses to a wide range of prompts.
Limitations and capabilities of ChatGPT
ChatGPT is a highly advanced language model, but it is not perfect. It is still limited by the biases and limitations present in the training data it was trained on. Additionally, ChatGPT may not always generate accurate or appropriate responses to certain prompts, especially if the input is unclear or if the context is missing. It is important to keep these limitations in mind when using ChatGPT and to carefully craft prompts that will maximize its performance.
For an overview of ChatGPT: Click Here
Factors affecting ChatGPT performance
The performance of ChatGPT is influenced by a variety of factors, including the context of the input, the length and complexity of the input, previous input and output, and the tone and language preferences of the user. In order to maximize the performance of ChatGPT, it is important to understand these factors and how they can impact the output generated by the model.
Contextual bias
Contextual bias refers to the ways in which the model’s outputs are influenced by the context in which it is used. For example, if the model is trained on a large corpus of text that contains biased language or perspectives, this bias will be reflected in its outputs. To minimize contextual bias, it is important to carefully curate the training data and ensure that it is diverse and representative of a wide range of perspectives and experiences.
Input length and complexity
The length and complexity of the input can also affect the performance of ChatGPT. Generally speaking, longer and more complex inputs can be more challenging for the model to process and may result in lower quality outputs. On the other hand, shorter and simpler inputs may be more straightforward for the model to process but may not provide enough context or information for the model to generate a meaningful response. It is important to strike a balance between input length and complexity to ensure that the model is generating high quality outputs.
Previous input and output
The previous input and output can also impact the performance of ChatGPT. When the model is generating a response, it takes into account the previous input and output in order to generate a coherent and contextually appropriate response. This means that if the previous input or output contains errors or is not relevant to the current conversation, it can impact the quality of the model’s response. To minimize this effect, it is important to ensure that the previous input and output are relevant, accurate, and appropriate for the current conversation.
User tone and language
Finally, the tone and language preferences of the user can also impact the performance of ChatGPT. A user who is using formal, technical language may expect different responses from the model than a user who is using casual, colloquial language. Understanding the tone and language preferences of the user is an important aspect of prompt engineering and can help to ensure that the model is generating responses that are appropriate and relevant to the user’s needs.
For example, a user who is looking for information about a technical topic may prefer a response that is written in a formal, technical style, while a user who is looking for information about a more casual or personal topic may prefer a response that is written in a more conversational style. By understanding the tone and language preferences of the user, you can craft prompts that are more likely to result in responses that are relevant, accurate, and appropriate for the user’s needs.
There are a variety of factors that can impact the performance of ChatGPT, including contextual bias, input length and complexity, previous input and output, and user tone and language preferences. By understanding these factors and incorporating them into your prompt engineering strategy, you can maximize the performance of ChatGPT and generate high quality outputs that are aligned with the user’s needs.
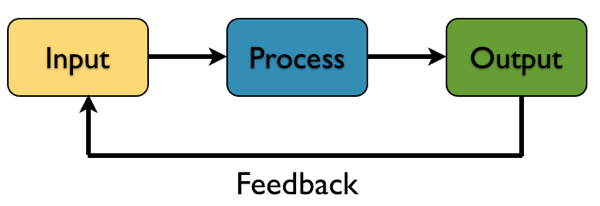
Techniques for effective prompt engineering
Prompt engineering is a critical aspect of maximizing the performance of ChatGPT. By carefully crafting prompts that are clear, concise, and aligned with the user’s needs, you can ensure that the model is generating high quality outputs that are relevant and accurate. In this section, we will explore some of the most effective techniques for prompt engineering and provide examples to illustrate their application.
Defining clear and concise prompts
Defining clear and concise prompts is one of the most important techniques for prompt engineering. The prompt serves as the foundation for the conversation between the user and the model, and it is important that it provides enough information to guide the conversation in a meaningful and productive direction.
For example, consider the prompt: “What is the best way to reduce carbon emissions?” This prompt is clear, concise, and provides enough information to guide the conversation in a productive direction. The model will be able to generate a response that is relevant and accurate based on the information provided in the prompt.
Utilizing context to improve accuracy
Utilizing context to improve accuracy is another important technique for prompt engineering. The more context you provide in the prompt, the more likely it is that the model will generate a response that is relevant and accurate.
For example, consider the prompt: “What is the best way to reduce carbon emissions in the transportation sector?” This prompt provides more context than the previous example and allows the model to generate a response that is more focused and specific. The model will be able to generate a response that is tailored to the transportation sector, which will be more relevant and accurate for the user.
Minimizing input length without sacrificing meaning
Minimizing input length without sacrificing meaning is another important technique for prompt engineering. By minimizing the length of the input, you can reduce the computational resources required to process the input, which can result in faster response times and improved performance.
For example, consider the prompt: “Reduce carbon emissions in transportation?” This prompt is shorter and more concise than the previous examples, but still provides enough information to guide the conversation in a meaningful and productive direction. The model will be able to generate a response that is relevant and accurate based on the information provided in the prompt, but with a lower computational cost, which will result in a faster response time and improved performance.
Managing user tone and language
Managing user tone and language is another important technique for prompt engineering. By understanding the tone and language preferences of the user, you can craft prompts that are more likely to result in responses that are relevant, accurate, and appropriate for the user’s needs.
For example, consider the prompt: “What is the most effective strategy for reducing carbon emissions in the transportation sector in a way that is sensitive to local communities and the environment?” This prompt incorporates the user’s tone and language preferences by considering the impact of the strategy on local communities and the environment, which will result in a response that is more aligned with the user’s needs.
Incorporating feedback and adjusting prompts accordingly
Incorporating feedback and adjusting prompts accordingly is the final technique for prompt engineering. By incorporating feedback from users, you can identify areas for improvement and adjust your prompts accordingly.
For example, if a user provides feedback that the responses generated by the model are not relevant or accurate, you can review the prompt and make adjustments to ensure that it is clearer, more concise, or provides more context as needed. By continuously incorporating feedback and adjusting your prompts, you can continuously improve the performance of the model and ensure that it is generating high quality outputs that are aligned with the user’s needs.
There are several effective techniques for prompt engineering, including defining clear and concise prompts, utilizing context to improve accuracy, minimizing input length without sacrificing meaning, managing user tone and language, incorporating feedback, and adjusting prompts accordingly. By incorporating these techniques into your prompt engineering strategy, you can maximize the performance of ChatGPT and generate high quality outputs that are aligned with the user’s needs.
Examples of effective prompt engineering
There are many real-life use cases of prompt engineering that demonstrate the effectiveness of this technique in maximizing the performance of ChatGPT. In this section, we will examine some of these use cases and analyze their success and what made them effective.
Real-life use cases of prompt engineering
One of the most well-known use cases of prompt engineering is the customer service chatbot. Many organizations use customer service chatbots to provide quick and efficient support to customers. These chatbots are trained on a large dataset of customer inquiries and are designed to respond to a wide range of customer questions.
Another example is the use of ChatGPT in healthcare. Healthcare organizations can use ChatGPT to help answer patient questions and provide information on health and wellness topics. For example, a healthcare provider might use a prompt such as: “What are the symptoms of a common cold?” to provide accurate and up-to-date information on this topic.
Another use case is in the financial industry, where ChatGPT can be used to provide financial advice and guidance to clients. For example, a financial advisor might use a prompt such as: “What are the best savings options for someone who wants to save for a down payment on a house?” to provide relevant and accurate information to clients.
Analyzing their success and what made them effective
The success of these use cases can be attributed to several factors. First and foremost, the prompts used in these use cases were clear and concise, providing the model with the necessary information to generate a relevant and accurate response. Additionally, the use of context was also critical in ensuring that the responses generated by the model were relevant to the user’s needs.
Another factor that contributed to their success was the use of a large dataset to train the model. By training the model on a large dataset, the model was able to learn from a diverse range of inputs and outputs, which helped to reduce contextual bias and improve accuracy.
Finally, the continuous incorporation of feedback and the ability to adjust prompts accordingly also played a key role in their success. By incorporating feedback from users and adjusting the prompts as needed, the performance of the model was able to continuously improve over time.
These real-life use cases demonstrate the effectiveness of prompt engineering in maximizing the performance of ChatGPT. By using clear and concise prompts, utilizing context, training the model on a large dataset, and incorporating feedback, organizations can generate high quality outputs that are aligned with the user’s needs.
For more information on real-life applications of ChatGPT, Click Here
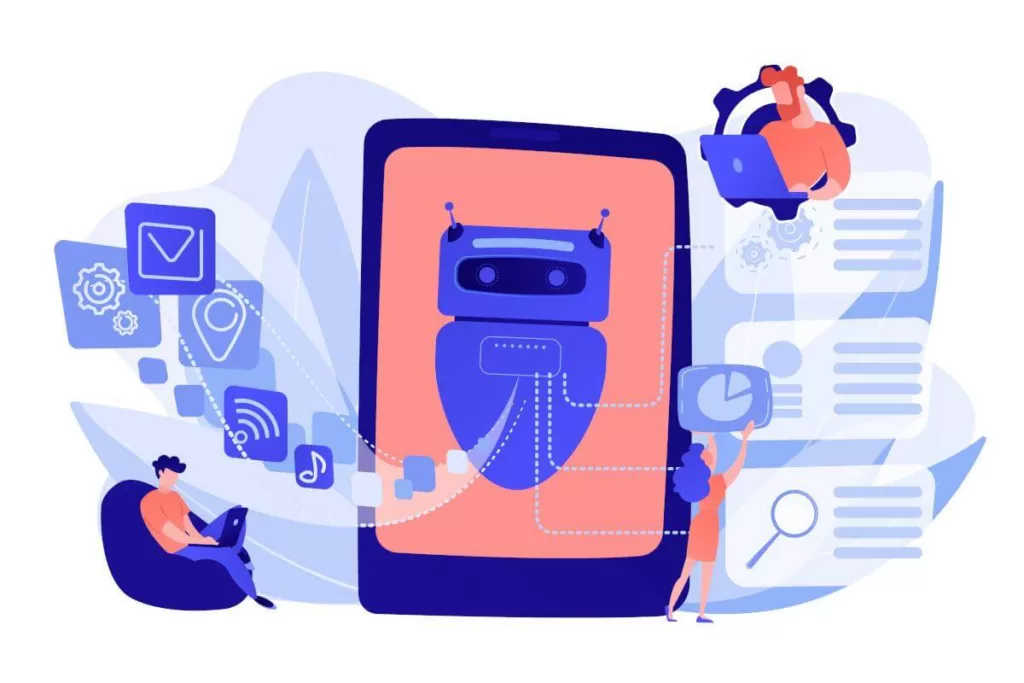
Challenges and considerations in prompt engineering
Prompt engineering can be a powerful tool for maximizing the performance of ChatGPT, but it also presents several challenges and considerations that must be taken into account. In this section, we will discuss some of the key challenges and considerations that organizations must keep in mind when using prompt engineering.
Balancing accuracy and natural language
One of the main challenges in prompt engineering is balancing accuracy and natural language. On the one hand, it is important to provide the model with clear and concise prompts that allow it to generate accurate and relevant outputs. On the other hand, it is also important to ensure that the model generates outputs that are natural and easy to understand for the user.
This can be a difficult balance to strike, as overly specific prompts can lead to robotic and unnatural outputs, while overly flexible prompts can result in responses that are vague or unhelpful. As a result, organizations must carefully consider the level of specificity they use when engineering prompts, and strive to find a balance that allows the model to generate accurate and natural outputs.
Addressing ethical and privacy concerns
Another important consideration in prompt engineering is addressing ethical and privacy concerns. As ChatGPT is trained on large datasets, it may be exposed to sensitive or personal information that could have serious consequences if misused.
Organizations must take steps to ensure that their models are properly trained and that the data used to train them is properly secured. This may involve implementing data privacy policies and security measures, as well as regular audits to ensure that the model is not being exposed to sensitive or personal information.
Navigating the trade-off between specific and flexible prompts
Finally, organizations must navigate the trade-off between using specific and flexible prompts. Specific prompts provide the model with a clear and focused input, which can result in accurate and relevant outputs. However, these prompts may not be flexible enough to handle a wide range of user inputs and may result in outputs that are not aligned with the user’s needs.
Flexible prompts, on the other hand, provide the model with a more open-ended input, which can result in outputs that are more natural and aligned with the user’s needs. However, these prompts may not be specific enough to generate accurate and relevant outputs and may result in outputs that are vague or unhelpful.
Navigating this trade-off can be challenging, and organizations must carefully consider the specific needs and requirements of their use case when engineering prompts. By striking a balance between specific and flexible prompts, organizations can maximize the performance of ChatGPT and provide users with the high-quality outputs they need.
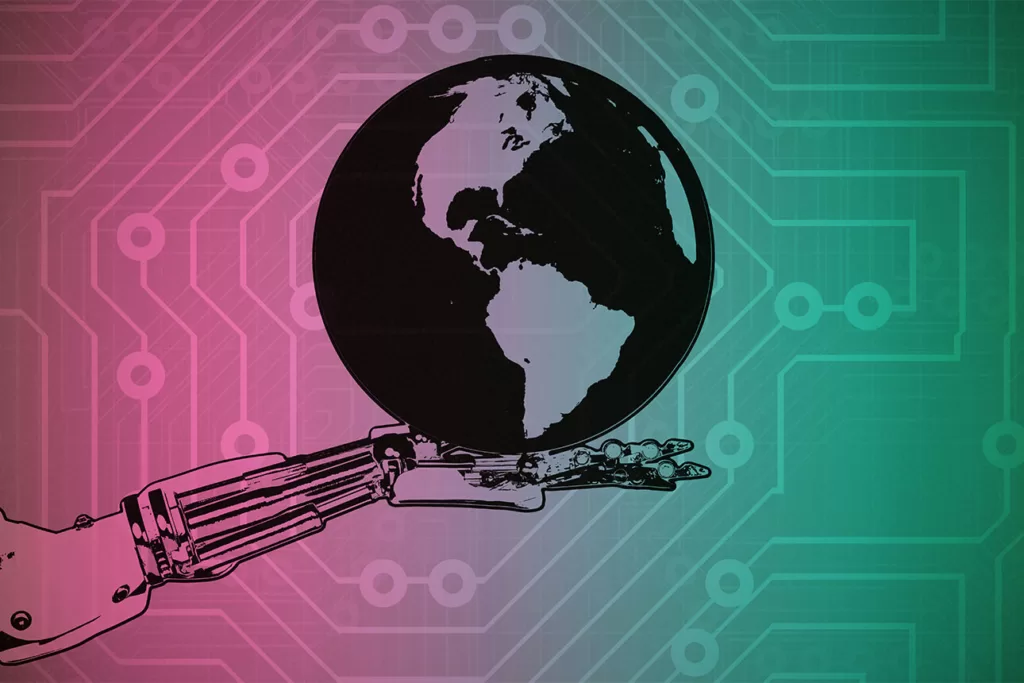
Conclusion
In this article, we have explored the role of prompt engineering in maximizing the performance of ChatGPT and the techniques and considerations that organizations must keep in mind when using this tool. We have discussed the factors affecting ChatGPT performance, including contextual bias, input length and complexity, previous input and output, and user tone and language. We have also outlined the techniques for prompt engineering, including defining clear and concise prompts, utilizing context to improve accuracy, minimizing input length without sacrificing meaning, managing user tone and language, and incorporating feedback and adjusting prompts accordingly.
Summary of key points
- Prompt engineering is a key tool for maximizing the performance of ChatGPT.
- The factors affecting ChatGPT performance include contextual bias, input length and complexity, previous input and output, and user tone and language.
- Techniques for prompt engineering include defining clear and concise prompts, utilizing context to improve accuracy, minimizing input length without sacrificing meaning, managing user tone and language, and incorporating feedback and adjusting prompts accordingly.
- Challenges and considerations in prompt engineering include balancing accuracy and natural language, addressing ethical and privacy concerns, and navigating the trade-off between specific and flexible prompts.
Final thoughts and recommendations for prompt engineering
Prompt engineering is a critical tool for organizations looking to maximize the performance of ChatGPT. By understanding the factors affecting ChatGPT performance and using effective techniques for prompt engineering, organizations can ensure that their models are generating high-quality outputs that are aligned with the needs and requirements of their users.
However, prompt engineering is not without its challenges and considerations, and organizations must carefully balance accuracy and natural language, address ethical and privacy concerns, and navigate the trade-off between specific and flexible prompts.
To get the most out of ChatGPT, organizations should approach prompt engineering with a strategic and data-driven approach, leveraging best practices and constantly monitoring and adjusting their prompts to ensure they are getting the best possible results. By doing so, they can unlock the full potential of this powerful tool and provide users with the high-quality outputs they need.